1. He has learned to take pride in competency and the effort it took him to get there. This shows in reading, playing piano, taekwondo, building things (LEGO and wood), helping with cooking and chores around the house, and most facets of life.
2. While we are reserving judgment on how smart he is (ok, we are pushing him into Kindergarten ahead of schedule, but that as much a result of the fact that preschools advance kids on their birthdays while the public school system advances people in cohorts as anything else (i.e. he has been in pre-K this past year since his birthday, but the standard public school timeline would have him repeating a year before he is on their timeline)), he does have an attention span and a pretty good level of perseverance (for a 4-year old). And we think the fact that he has the attitude that it is good to work hard to do something difficult as he starts his school years is a success on our end.
3. We have had guests with us this week. One of their comments was he seems like he is the type of kid who will never complain about things being unfair because he did not get something. We are pretty sure that he has learned to value people over things (although, if the things in question are books, it is a close call), And I think that half of the attraction of usual rewards like candy, toys, and trophies is because he gets from others that he is supposed to like it. (we occasionally have candy laying out, and don't realize there is an issue until other kids come into the house, because T won't go after them)
4. He likes helping around the house. Actually, when he makes a mess, he tries to clean up before we find out about it. It is really funny when he needs some supplies to do it. Last week he was asking me to help him get paper towels, "but don't come downstairs to look at the table"
5. He approaches the world engaged and looking around. We see that in his visits to museums, when we go on walks in parks and trails, and especially in taekwondo (my impression that this is the biggest differentiater among the pre-schoolers is their ability to focus on the teachers and class.
6. He is generally happy. The main testimonials are a couple kids that are in both his pre-school and his taekwondo school who have stated that "T is always happy"
7. He plays with other kids. This was a bit of a concern up to when he was around 3 1/2 or so. One thing that helps is there are a number of kids just a little older than him on our street (mostly girls). Now, the preschool teachers say that our little turtle is out of his shell and they have to tell him to stop talking. One of the joys about taking him to taekwondo is that we get to watch him interact with the other kids. And while we don't encourage it, he interacts with all of the other kids as they are waiting their turns for drills and such. As one of the smallest kids in the school (I think he is now the second smallest, one of the owners refers to him as one of their peanuts), We don't ever see him being one of the really talkative kids in his class and we expect that he will always take time to get used to new adults (neither he nor his sister were ever pass around kids),
8. He is learning how to present what he knows. Since we actually talk about what is going on at the museum or science center, we have been having him show the other kids and parents (advantage of having academics as parents, we actually know more than the kids volunteering there about whatever we are looking at, most of the time). These past few months he has been getting markably better at it.
9. He adores his little sister. (and she does likewise). From day 1 he has enjoyed being the big brother and getting to play and help care for his baby sister.
10. He still does not sleep on his own. Back when he was a baby with colic, the pediatrician warned us that he probably would not sleep on his own until he was four. Well, he is four, what's up (jk) At this point he can sleep by himself and does occasionally, but he really likes to check every now and then that someone is around. And he likes the snuggle. And, well, since I can pretty much sleep through the occasional check-in it does not bother me so why not. And I'm sure that there will be an end to this.
11. He is very careful. In some sense this is good. There are a lot of things we never had to worry about. He tested things before trying to eat them, We never really needed baby gates with him (because he figured out how to go backwards down before he started getting to fast for us to catch him), we can let him cut food with a (plastic) knife. We probably did not do a complete job on babyproofing things. But this goes along with not being daring in trying new things, until we go through with him first.
12. Not very creative. This somewhat goes with being careful, but he never had the phase of coloring and drawing with abandon. So we went from scribbling to deliberate outlining shapes, but not the randomish stick drawings that are stereotypical of pre-schoolers. I've started him on learning to draw using primitives (assembling basic shapes until they look like something) but the wild abandon that many kids keep through early elementary school never took with him.
13. He is no longer the the island of stillness in the chaos of toddler daycare like when he was 3, but he still tends to focus on one thing for extended periods of time, even as all of his peers fly from task to task.
14. He is becoming more expressive. With people he knows, he is willing to express himself.
15. In Fate Approaches terms, he would be Good at Careful, Fair at Quick and Clever, Average at Forceful and Flashy, and Poor at Sneaky.
16. We pretty much skipped tantrums. He has occasional crying spells, but his tantrums seem like he is trying to imitate what he thinks a tantrum should look like.
17. He has little sense of possessiveness. His daycare teachers have commented he does not fight over toys, because he does not have that sense of possessiveness and greed. He has more of a sense that something is his, but it still is not that strong.
18. He is pretty much what you see is what you get. No deceptiveness at all. Well, he is in the stage of saying what he thinks we want to hear (which would be considered lying if he were older), but even that is not that bad (exhibit: saying "I need a paper towel. But don't come downstairs, there is no mess")
Now we are getting ready for Kindergarten. We are going to a local catholic school, because they are the only ones who would consider taking in someone early (with recommendations from his pre-K teachers and an assessment, of course). They are going to get a kid who reads Dr. Seuss before entry into Kindergarten. Judging by their reactions, they can handle a wide range in a Kindergarten class. We have found out in his daycare/pre-school that T responds well in settings with small sizes (also helped a lot in taekwondo) and we are looking forward to his starting school.
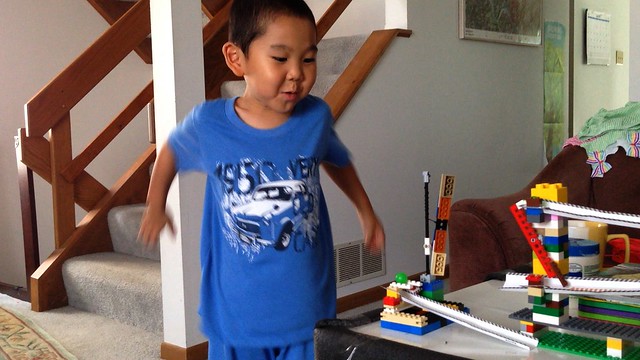